How Biases in Artificial Intelligence Impact Global Health
- Pre-Collegiate Global Health Review
- Nov 18, 2023
- 4 min read
By Eera Bhatt, Troy High School, Troy, Michigan, USA
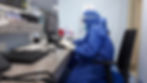
Summary
During the COVID-19 pandemic, significant advancements have been made in the field of artificial intelligence (AI) concerning disease detection and diagnosis. Nevertheless, even trained AI systems have reflected human biases and produced prejudiced results that have been inaccurate and dangerous for certain groups of patients. Especially due to the increased usage of AI for global health studies and medical diagnoses, recent efforts have been put forth to reduce its biases by diversifying software teams and involving clinicians in the AI modeling process.
Technology in the Healthcare Industry
In the field of epidemiology, traditional public health surveillance systems have been primarily based on medical statistics. However, AI has enhanced such systems as it can also analyze unstructured medical data such as text, images, videos, clinical notes, and information from social media—types of data with no pre-defined format. The usage of multidisciplinary data—data collected from various sources—has enabled AI to make accurate and efficient analyses of disease transmission using machine learning (Zeng et al., 2020). Machine learning (ML) is a branch of artificial intelligence in which a computer model trains itself with data to identify patterns or predict future outcomes based on past examples (Brown, 2021). Alongside additional subcategories of AI, ML utilizes multiple data sources to perform tasks such as accurately and efficiently predicting trends in the spread of disease and detecting disease outbreaks (Figure 1).

Figure 1: Methods by which AI enhances existing public health surveillance and response approaches (Zeng et al., 2020).
However, biases can arise in AI healthcare models from discriminatory or incomplete data, existing global inequities influencing the data samples, and prejudiced model building practices. For instance, Caucasians constitute approximately 80% of genomics and genetics data, meaning certain medical studies may be more applicable to Caucasians than to underrepresented groups (Igoe, 2021). Biased ML predictions may have multiple causes such as an unfair data collection process, imbalanced gender-based or racial classes of data in the model training stage, and human biases that adversely impact the computer model (Figure 2).

Figure 2: Common sources of bias in AI that impact medical projects (Norori et al., 2021).
A landmark study involved a clinical algorithm that was implemented by multiple hospitals to determine which ill patients required extra care. According to the AI model’s results, black patients had to be much more diseased than white patients to be recommended for an equivalent amount of medical care. A racial bias occurred since the clinical algorithm was trained on older data that was related to health costs. The outdated health cost data reflected a history in which black patients spent less money on their healthcare than whites did due to former income and wealth disparities and previously, an unequal access to medical care. After fixing the data’s racial disparity, the researchers of the study saw the percentage of black patients requiring additional care increase from 17.7% to 46.5% (Obermeyer et al., 2019).
Software developers are often excessively focused on achieving high overall accuracies for the patients in their AI models’ prediction results. However, a high overall accuracy might be inequitable for certain minorities of patients that are scarcely present in the data if present at all. This causes the AI to display biases against such minorities that are especially damaging in a global health context (Igoe, 2021). Biases may appear in almost any stage of an experiment and must be checked for during every step of the ML process.
One way to combat medical biases in AI is for data science teams to recruit workers who have diverse racial and ethnic backgrounds as this accounts for several perspectives in the algorithm development stages (Igoe, 2021). Diverse developer teams are more familiar with the typical challenges that are faced by underrepresented groups and minorities in clinical datasets. Additionally, adding clinicians to data science teams can alleviate ML prejudices, as medical workers can offer clinical insight into the processes that create the data (Panch et al., 2019). Clinicians can also implement feature engineering which involves the creation of new features (patient’s age, patient’s gender, etc.) based on the raw data to improve the AI model’s performance. For instance, if a clinician deems the patient’s age an important factor in the medical diagnosis or study, the data samples can be grouped by age to enhance the model’s results (Roe et al., 2020). The process of feature engineering cleans up the format of the data enabling the clinical algorithm to operate with ease (Heaton, 2016). For instance, various gender-based, ethnic, or racial groups may require different clinical variables or characteristics to be measured for each group in a dataset. To account for this, developers often need multidisciplinary data for clinical projects.
In the context of global health, it is important to recognize that a universally applicable AI model is unlikely due to the limited availability of clinical data for every socioeconomic group. This means that certain classes will be scarcely sampled in datasets used for training AI models (Panch et al., 2019). Additionally, a certain amount of clinical AI bias will always exist since the inequities behind bias already influence those who build the computer algorithms (Obermeyer et al., 2019).
Currently, however, several health professionals do understand the prevalence of AI bias and its adverse impact on global health studies (Igoe, 2021). Multiple technology and data science companies are actively promoting diversity, equity, and inclusion (DEI) in their teams to combat potential prejudices against underrepresented groups of patients in studies. While medical bias in AI cannot be completely eliminated, health professionals and developers must work together to minimize these biases and ensure that minorities receive adequate medical care.
References