Saketh Sundar, River Hill High School, Clarksville, Maryland, USA
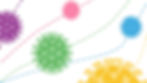
Throughout the COVID-19 pandemic, headlines ranging from “Coronavirus forecasts are grim: It’s going to get worse” to “Covid-19 cases and deaths in the US will fall over the next four weeks, forecast predicts” have dominated the news (Achenbach, 2020; Kallingal, 2021). The weekly-published Center for Disease Control and Prevention (CDC) COVID-19 forecasts have become the go-to forecasts for the media, the public, and various levels of government (Cramer et al., 2021). These projections, generated from epidemiological forecasting, not only inform the public’s caution towards the pandemic but are also crucial for officials to create public health guidelines and allocate resources in hospitals (Gibson et al., 2020). But where do these predictions come from?
Epidemiological forecasting is not a new field of study. Scientists have used mathematical and statistical models to predict the spread of infectious diseases since the 1920s (Brauer et al., 2012). They are still regularly used for endemics, such as to predict the spread and mortality of influenza throughout the yearly flu season (McGowan et al., 2019). However, during the COVID-19 pandemic, not only did the field rise to the spotlight, but it became instrumental in informing the world about the trajectory of the pandemic.
The CDC forecasts are generated by the COVID-19 Forecast Hub, which consists of dozens of modeling teams across the globe. After each modeling team submits its own forecast, they are all used to build a single ensemble forecast that is disseminated to the public by the CDC (Ray et al., 2020). The forecasts submitted to the COVID-19 forecast hub are not just guesses made by experts but are generated by mathematical, statistical, or machine learning (a branch of artificial intelligence) models (Cramer et al., 2021). Creating a model to forecast a pandemic, however, is no easy task. Regardless of the approach to modeling, multiple factors such as the lack of data availability, inconsistencies in reporting, and a lack of knowledge about the pathogen in the early stages of the pandemic have made forecasting the COVID-19 pandemic incredibly difficult (Koerth, 2020). Likewise, forecasting in developing and low-income countries is an immense challenge. The issues illustrated above are exacerbated by the countries’ lack of robust public health infrastructure and data sparsity across multiple data sources (Deen et al., 2014).

Figure 1. The national forecast of COVID-19 deaths for the week of June 21, 2021 (Center for Disease Control and Prevention, 2021).
There are many types of modeling within epidemiological forecasting, but most models can be classified into mechanistic and non-mechanistic models (Brooks et al., 2018). An example of a mechanistic model is the SEIR (Susceptible, Exposed, Infectious, Recovered) which uses information about the mechanism of the pathogen and population to predict the future spread and mortality of the disease (Carcione et al., 2020). In contrast, non-mechanistic models learn from previous trends in data without needing structure, which make them more flexible to fit the available data. Non-mechanistic models can be implemented using traditional statistical methods or machine learning (a branch of artificial intelligence) (Brooks et al., 2018).
There are pros and cons to each type of model. Though non-mechanistic models are usually more flexible, they are limited in guiding public health policy because they lack a framework for causal inference, which allows researchers to draw causal conclusions based on data (Baker et al., 2018) to evaluate the effectiveness of policies. Mechanistic models have numerous challenges to accurately forecast mortality, including the discrepancy between patient-level clinical information and population-level modeling, inadequate inclusion of individual behavioral and social influence, and little flexibility for new evidence (Chen et al., 2020). A benefit of these models, however, is that they can generate multiple simulations of various scenarios and factors to predict different hypothetical situations (Campaner, 2011). Since mechanistic models can produce forecasts of alternative realities, they can inform preventative public health policies and interventions to reduce overall transmission.
The emergence of machine learning, as well as newer statistical methods in recent decades, has made forecasting an easier and more accurate task (Makridakis et al., 2018). There is no doubt that epidemiological forecasting will sustain its relevance in years to come, as scientists predict an increase in the prevalence of epidemics and pandemics in the future. Next time you see a forecast for COVID-19 or a future pandemic, you will now be informed of where these predictions come from and have more faith in them.
References
Achenbach, J. (2020). Coronavirus forecasts are grim: ‘It’s going to get worse.’ The Washington Post.https://www.washingtonpost.com/health/coronavirus-forecasts-are-grim-its-going-to-get-worse/2020/03/11/2a177e0a-63b4-11ea-acca-80c22bbee96f_story.html
Baker, R. E., Peña, J.-M., Jayamohan, J., & Jérusalem, A. (2018). Mechanistic models versus machine learning, a fight worth fighting for the biological community? Biology Letters, 14(5), 20170660. https://doi.org/10.1098/rsbl.2017.0660
Brooks, L. C., Farrow, D. C., Hyun, S., Tibshirani, R. J., & Rosenfeld, R. (2018). Nonmechanistic forecasts of seasonal influenza with iterative one-week-ahead distributions. PLOS Computational Biology, 14(6), e1006134. https://doi.org/10.1371/journal.pcbi.1006134
Brauer, F., & Castillo-Chavez, C. (2012). Epidemic models. In F. Brauer & C. Castillo-Chavez (Eds.), Mathematical Models in Population Biology and Epidemiology (pp. 345–409). Springer. https://doi.org/10.1007/978-1-4614-1686-9_9
Carcione, J. M., Santos, J. E., Bagaini, C., & Ba, J. (2020). A simulation of a COVID-19 epidemic based on a deterministic SEIR model. Frontiers in Public Health, 8. https://doi.org/10.3389/fpubh.2020.00230
Campaner R. (2011). Mechanistic causality and counterfactual-manipulative causality: recent insights from philosophy of science. Journal of epidemiology and community health, 65(12), 1070–1074. https://doi.org/10.1136/jech.2011.134205
Chen, S., Robinson, P., Janies, D., & Dulin, M. (2020). Four Challenges Associated With Current Mathematical Modeling Paradigm of Infectious Diseases and Call for a Shift. Open Forum Infectious Diseases, 7(8). https://doi.org/10.1093/ofid/ofaa333
Cramer, E. Y., Ray, E. L., Lopez, V. K., Bracher, J., Brennen, A., Rivadeneira, A. J. C., Gerding, A., Gneiting, T., House, K. H., Huang, Y., Jayawardena, D., Kanji, A. H., Khandelwal, A., Le, K., Mühlemann, A., Niemi, J., Shah, A., Stark, A., Wang, Y., … Reich, N. G. (2021). Evaluation of individual and ensemble probabilistic forecasts of COVID-19 mortality in the US. MedRxiv, 2021.02.03.21250974. https://doi.org/10.1101/2021.02.03.21250974
Deen, J., Von Seidlein, L., & Clemens, J. D. (2014). Issues and challenges of public-health research in developing countries. Manson’s Tropical Infectious Diseases, 40-48.e1. https://doi.org/10.1016/B978-0-7020-5101-2.00006-6
Gibson, G. C., Reich, N. G., & Sheldon, D. (2020). REAL-TIME MECHANISTIC BAYESIAN FORECASTS OF COVID-19 MORTALITY. Cold Spring Harbor Laboratory Press. https://doi.org/10.1101/2020.12.22.20248736
Kallingal, M. (2021, May 19). Covid-19 cases and deaths in the US will fall over the next four weeks, forecast predicts. CNN Health. https://www.cnn.com/2021/05/28/health/us-coronavirus-friday/index.html
Koerth, M. (2020, March 31). Why it’s so freaking hard to make a good covid-19 model. FiveThirtyEight. https://fivethirtyeight.com/features/why-its-so-freaking-hard-to-make-a-good-covid-19-model/
Makridakis, S., Spiliotis, E., & Assimakopoulos, V. (2018). Statistical and Machine Learning forecasting methods: Concerns and ways forward. PLOS ONE, 13(3), e0194889. https://doi.org/10.1371/journal.pone.0194889
National Forecast of New and Total COVID-19 Deaths [Chart]. (n.d.). Center for Disease Control and Prevention. https://www.cdc.gov/coronavirus/2019-ncov/science/forecasting/ forecasting-us.html
Ray, E. L., Wattanachit, N., Niemi, J., Kanji, A. H., House, K., Cramer, E. Y., Bracher, J., Zheng, A., Yamana, T. K., Xiong, X., Woody, S., Wang, Y., Wang, L., Walraven, R. L., Tomar, V., Sherratt, K., Sheldon, D., Reiner, R. C., Prakash, B. A., . . . Reich, N. G. (2020). Ensemble Forecasts of Coronavirus Disease 2019 (COVID-19) in the U.S. Cold Spring Harbor Laboratory Press. https://doi.org/10.1101/2020.08.19.20177493
The Influenza Forecasting Working Group, McGowan, C. J., Biggerstaff , M., Johansson, M., Apfeldorf, K. M., Ben-Nun, M., Brooks, L., Convertino, M., Erraguntla, M., Farrow, D. C., Freeze, J., Ghosh, S., Hyun, S., Kandula, S., Lega, J., Liu, Y., Michaud, N., Morita, H., Niemi, J., … Reed, C. (2019). Collaborative efforts to forecast seasonal influenza in the United States, 2015–2016. Scientific Reports, 9(1), 683. https://doi.org/10.1038/s41598-018-36361-9
Article Thumbnail: [An artistic visualization of coronavirus forecasts]. (2020). FiveThirtyEight.
https://fivethirtyeight.com/wp-content/uploads/2020/04/COVID-TRACKER_16X9.png