Diabetic Retinopathy Screening - The Rise of Artificial Intelligence in Healthcare
- Pre-Collegiate Global Health Review
- Dec 21, 2020
- 4 min read
Updated: Nov 22, 2021
By Aditya Syam, Jamnabai Narsee International School, Maharashtra, Mumbai, India

Artificial Intelligence (AI) based applications have already made inroads into many aspects of our lives. The global consultancy firm PwC estimates that AI will contribute $15.7 trillion USD to the global economy by 2030.
The PwC AI Impact Index, which identifies eight sectors with the biggest potential for AI impact, places healthcare right at the top amongst all these sectors (Sizing the Prize, PwC, 2017).
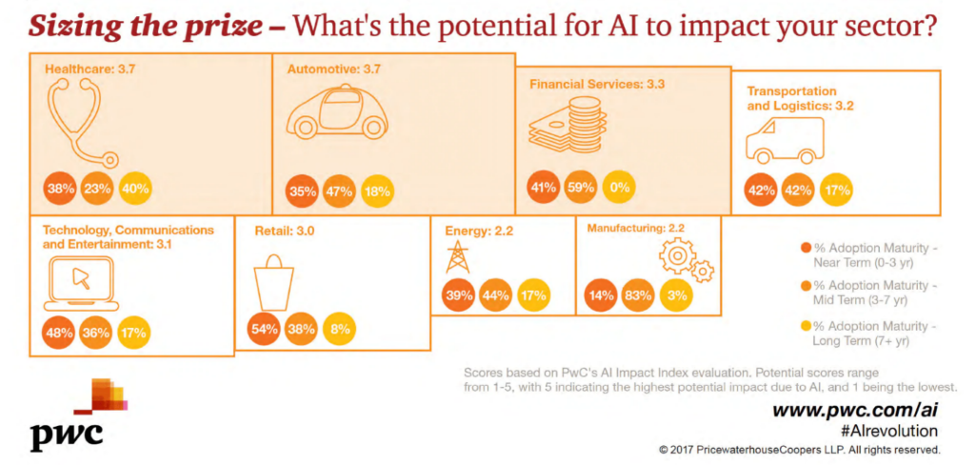
The first application of AI in healthcare was in 1964, with Eliza, the very first chatbot (Siwicki, 2020). Since then, AI-based applications have truly gained momentum on the back of developments in Deep Learning and Neural Networks.
The areas within healthcare where AI tools are currently being used can be divided into four broad categories – administrative services, screening & diagnosis, treatment, nursing & monitoring, and drug discovery & medicine management (Sinhasane, 2019).
One of the most exciting applications of AI has been in screening. The ability of deep learning models and neural networks to screen thousands of medical images in seconds is a powerful tool in widening the reach and accuracy of healthcare provision.
In fact, AI has already made great strides with regards to improving the quality of care for patients with diabetic retinopathy (DR) – a condition that affects the eyes of patients with type 1 or type 2 diabetes. It is caused due to damage to the blood vessels of the light-sensitive tissue at the back of the retina and can eventually lead to blindness – something that is completely preventable if detected in time (Diabetic retinopathy, 2018).
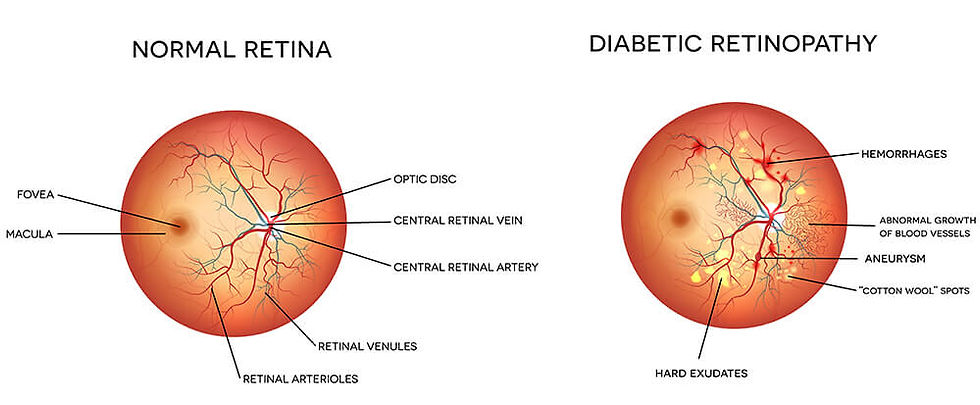
In 2015, Google and Verily started a pilot program with two well-known Indian eye hospitals (Aravind Eye Hospital and Shankara Nethralaya) to test AI-based applications in ophthalmology, which involved using AI-based tools to screen for DR. Google’s study indicated that about 45% of patients suffer vision loss before diagnosis.
The initial results were very promising, and the pilots have subsequently been scaled up (Bringing AI and machine learning innovations to healthcare (Google I/O '18), 2018).
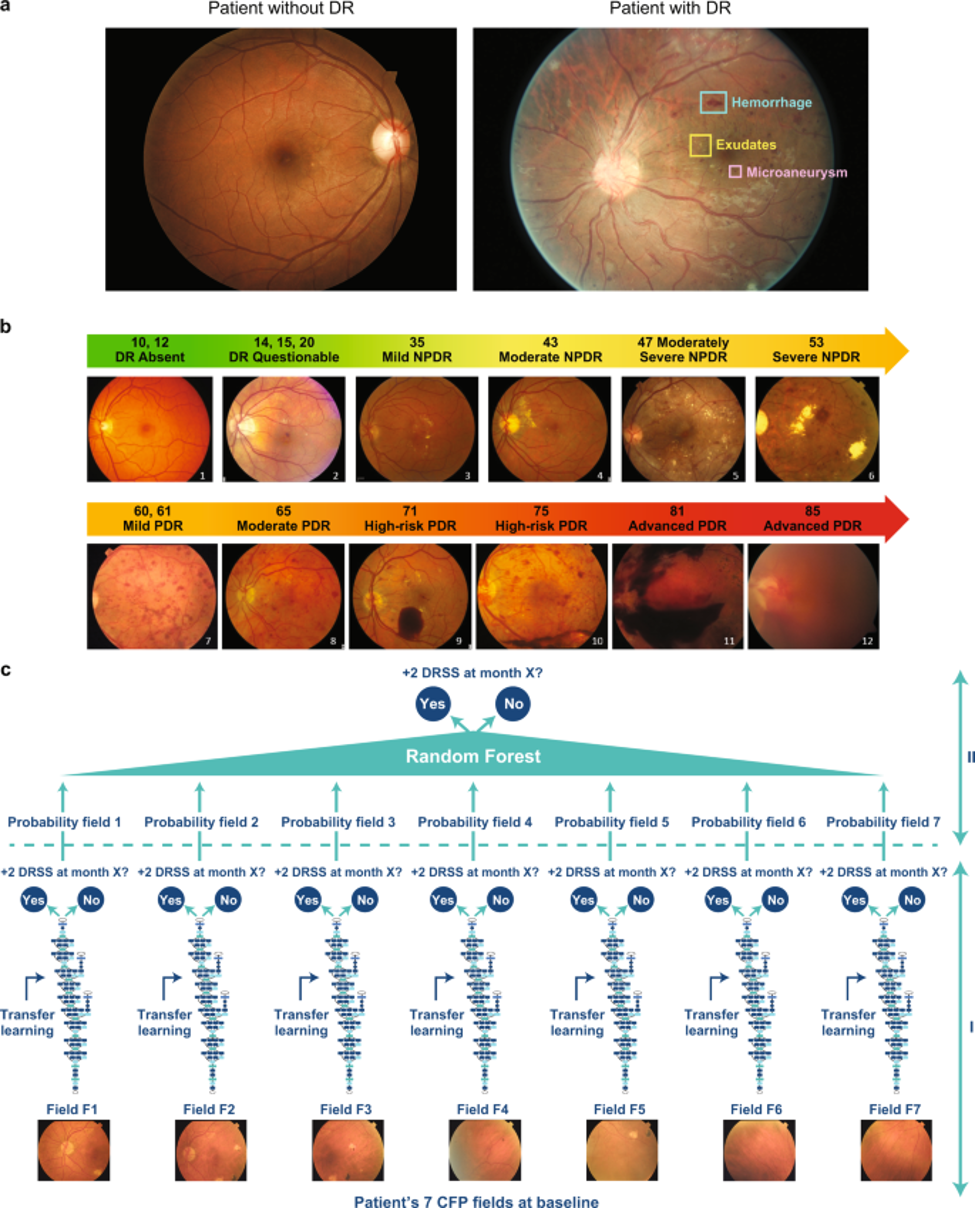
The picture above is a depiction of a deep learning model that is meant to detect the presence of DR.
AI applications enable screening at scale, addressing issues of both accessibility (image of patient’s retina is captured and sent electronically to AI-based screening machines) and affordability (machines can screen multiple images in the time taken by an eye doctor to screen one patient, thus bringing down per patient cost).
AI’s accuracy of diagnosis improved rapidly from being on par with ophthalmologists (December 2016) to being on par with retinal specialists (February 2018). The accuracy levels were high on both parameters – sensitivity (the ability of the test to correctly identify those with the disease, i.e. true positive rate) and specificity (the ability of the test to correctly identify those without the disease, i.e. true negative rate).
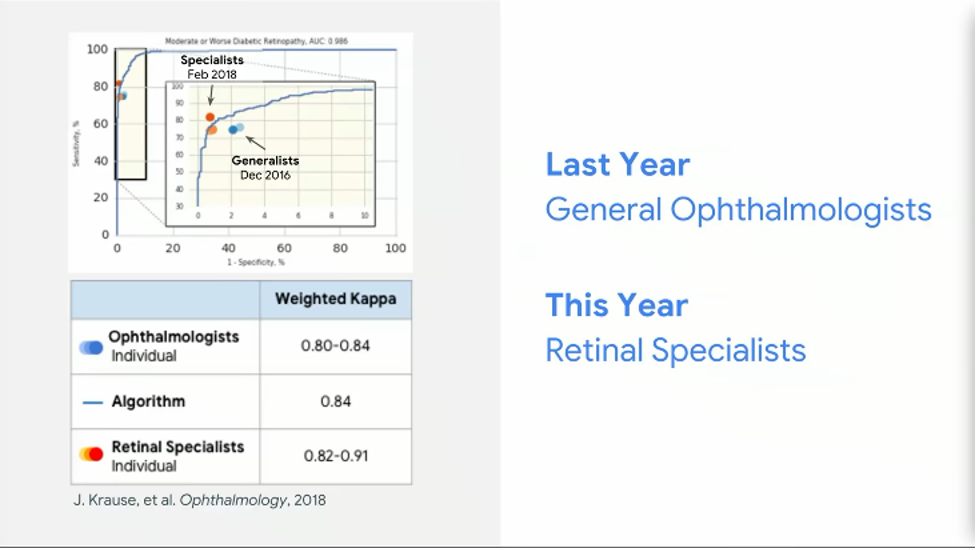
The graph above shows the accuracy of AI systems versus doctors in detecting Diabetic Retinopathy.
While the primary objective of Google’s pilot program was to analyse the efficacy of AI in screening for DR, the AI systems offered more insights into a seemingly unrelated field. The same retinal scans used for diagnosis of DR could now be used by the AI systems to predict the risk of an individual to have an adverse cardiovascular event – heart attack or stroke – over the subsequent 5-year period. Based on just a retinal scan, the AI system could predict parameters such as age, sex, smoking habits, blood glucose levels, body mass index, and blood pressure with a remarkably high degree of accuracy. This now opens the door to a new non-invasive method to detect cardiovascular risk (Sundar Pichai on the application of AI in healthcare (Google I/O 2018), 2018).
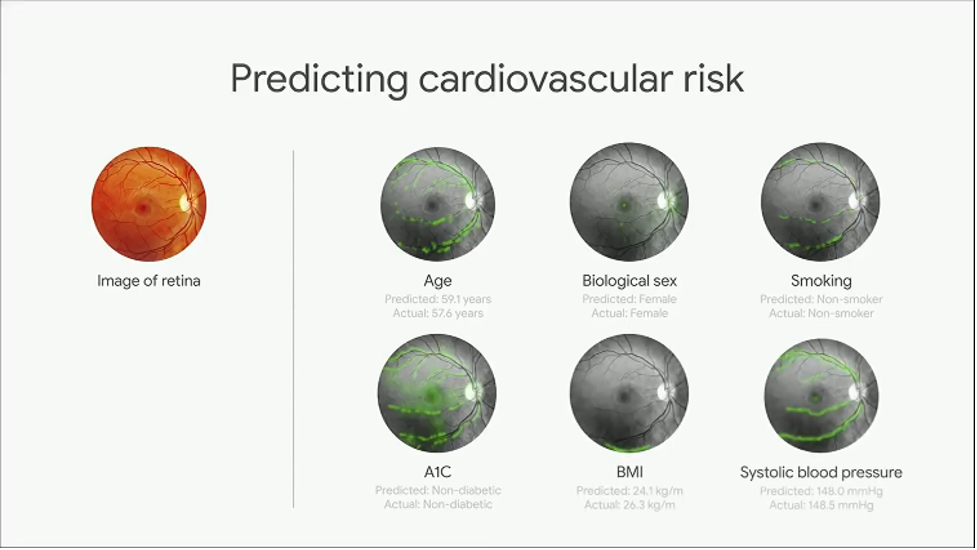
By bringing expert diagnosis to places where trained specialists are scarce, Google and Verily have demonstrated the ability to successfully implement AI-based applications to deliver healthcare service that is of a high quality, rapidly scalable, and cost effective.
However, concerns with AI applications include privacy and ethics (Mahapatra, 2019), biases and prejudices in the historical data used for training the algorithms (Harvard, 2019), and the threat of adversarial attacks which can give rise to rogue machines (Warnings of a Dark Side to A.I. in Health Care, NYT, 2019).
The use of AI in healthcare, nonetheless, can drastically change the current lack of healthcare access that plagues much of the world. Once an AI-based model is sufficiently trained, it needs minimal human monitoring and can constantly function at high levels of accuracy, without additional monetary resources, and in the absence of any fatigue. Since it is digital and can be remotely operated, it can be made much more accessible in areas which lack professionals.
All this combined with the wide range of AI applications is paving the way for the next revolution in the healthcare sector – the rise of Artificial Intelligence.
References
Google Developers. (2018). Bringing AI and machine learning innovations to healthcare (Google I/O '18). https://www.youtube.com/watch?v=JzB7yS9t1YE.
Harvard Medical School. (2019). Md vs. Machine: Artificial intelligence in health care. YouTube. https://www.youtube.com/watch?v=xSDfma4VEx8&feature=youtu.be.
Mahapatra, D. (2019, June 16). Overview of AI in Indian healthcare. AI4Bharat. https://ai4bharat.org/articles/overview-of-ai-in-indian-healthcare.
Mayo Foundation for Medical Education and Research. (2018, May 30). Diabetic retinopathy. Mayo Clinic. https://www.mayoclinic.org/diseases-conditions/diabetic-retinopathy/symptoms-causes/syc-20371611.
Metz, C., & Smith, C. S. (2019, March 21). Warnings of a Dark Side to A.I. in Health Care. The New York Times. https://www.nytimes.com/2019/03/21/science/health-medicine-artificial-intelligence.html.
npj | digital medicine. (2019). Deep learning algorithm predicts diabetic retinopathy progression in individual patients. photograph. https://www.nature.com/articles/s41746-019-0172-3.
PwC. (2017). PwC's Ai Index analyses the impact of Ai on sectors. AI to drive GDP gains of $15.7 trillion with productivity, personalisation improvements. photograph. https://www.pwc.com/gx/en/news-room/press-releases/2017/ai-to-drive-gdp-gains-of-15_7-trillion-with-productivity-personalisation-improvements.html.
PwC. (2017, June). What doctor? Why AI and robotics will define New Health.
PwC. Sizing the prize - What’s the real value of AI for your business and how can you capitalise?
qure.ai. Artificial Intelligence for Radiology. qure.ai. photograph. https://qure.ai/.
Sinhasane, S. (2020, November 23). Top 15 Promising AI Applications in Healthcare. Mobisoft Infotech. https://mobisoftinfotech.com/resources/blog/top-15-ai-applications-in-healthcare/.
Retina Charleston: Diabetic Retinopathy Charleston: Carolina Eyecare. (2019, October 17). Retrieved December 21, 2020, from https://www.carolinaeyecare.com/retina-charleston/diabetic-retinopathy/
Siwicki, B. (2020, June 11). The origins of AI in healthcare, and where it can help the industry now. Healthcare IT News. https://www.healthcareitnews.com/news/origins-ai-healthcare-and-where-it-can-help-industry-now.
Stanford Medicine. (2019). The state of artificial intelligence in medicine. YouTube. ttps://www.youtube.com/watch?v=PZQMyj-9z-w&feature=youtu.be.
The Opportunities & Challenges of AI In Healthcare. (2017, February 10). Retrieved from https://www.topbots.com/healthcare-ai-opportunities-challenges-policy-workflow/ (Originally photographed 2017, February 10).
YouTube. (2018). Sundar Pichai on the application of AI in healthcare (Google I/O 2018), 2018. https://www.youtube.com/watch?v=HYUhtnHJcNA.
Comments